
Researchers in Iran have used a deep neural network (DNN) to extract the foetal electrocardiogram (ECG) from a single abdominal ECG channel. Their method, described in Physiological Measurement, may improve foetal monitoring in the future.
How to isolate foetal ECG?
Currently, the electrical activity of a foetus’ heart is measured using an ECG acquired from electrode patches placed on the expectant mother’s abdomen. Clinicians can use the foetal ECG to evaluate foetal health and diagnose abnormalities.
The challenge? It’s difficult to isolate the foetal ECG signal from abdominal ECG because the latter contains signals from both the foetus (“foetal ECG”) and the mother (“maternal ECG”), as well as from sources of interference, such as muscle contractions. This task becomes even more demanding toward the end of pregnancy, when the amplitude of the foetal ECG signal is comparable to that of the maternal ECG.
Arash Rasti-Meymandi, lead author on the study and a graduate student at the Iran University of Science and Technology, and his colleagues thought of a potential solution to this problem that relies on DNNs.

Rasti-Meymandi was inspired by Unets, convolutional networks that are often used in medical image segmentation tasks. He and co-author Aboozar Ghaffari applied a modified version of a Unet to extract first the maternal ECG and then the foetal ECG signals.
“The Unet was able to outperform other techniques in image segmentation challenges,” Rasti-Meymandi says. “To extract different components of the abdominal ECG, we examined the abdominal ECG signal at different resolutions, [similar to the process used in a Unet model].”
The researchers’ DNN – called AECG-DecompNet – extracts the foetal ECG from a single channel of abdominal ECG using two sub-networks in series. The first sub-network extracts the maternal ECG signal; the second, the foetal ECG. The researchers trained the two sub-networks separately using simulated ECG signals and then evaluated the sub-networks using both simulated and real abdominal ECG recordings.
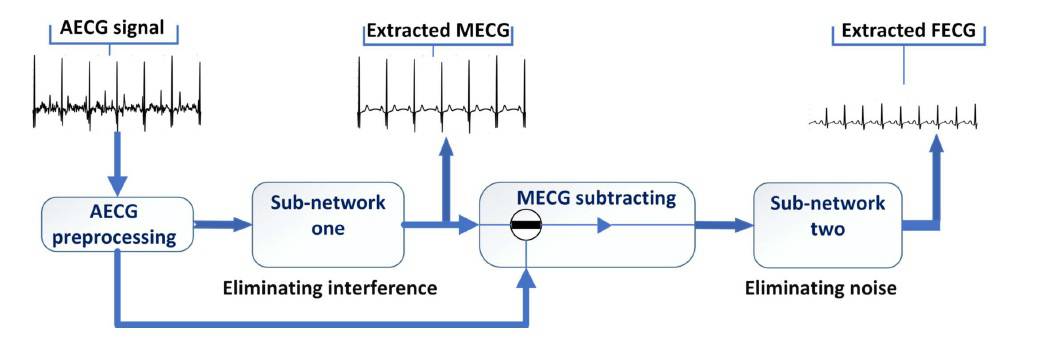
Using a graphics processing unit, the researchers’ DNN could process four seconds of abdominal ECG recording in approximately one second.
The future of DNNs and foetal ECG
Unlike other signal denoising methods, which require the morphology (P, Q, R, S and T waveforms indicating the heart’s electrical activity) of a reference ECG, multiple channels of ECG, or both, the researchers’ method requires only a single channel. This not only improves the mother’s comfort during ECG acquisition but also requires fewer resources and less time to implement than traditional ECG recording and signal extraction methods.
The researchers also found that relative to other methods, theirs better preserved the shape and structure of foetal ECG signals – all five waveforms were well-preserved for examination and diagnosis of foetal abnormalities.
“The primary result of this research is the effectiveness of using DNNs to extract foetal ECG signal from a single-channel abdominal recording with high precision,” Rasti-Meymandi tells Physics World. “We are currently working on a more sophisticated algorithm … to further increase the accuracy of the extracted heart rate.”
The team is also working on ways to implement their DNN in real-time on smartphones.
Limitations of their method include a potential overreliance on the training dataset, especially when the foetal ECG signal is weak, and the potential for errors to be propagated to the second sub-network from the first.