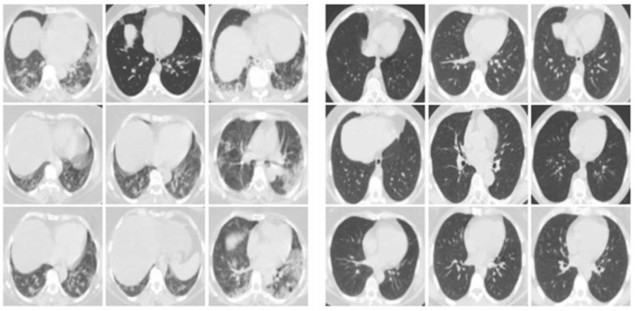
A new deep-learning framework to diagnose COVID-19 from patient lung scans – called KarNet – has been developed by researchers in India. The system, which uses transfer learning rather than needing a large training dataset, can achieve up to 97% accuracy in detecting SARS-CoV-2 infection.
As the coronavirus pandemic continues across the globe, faster and more reliable techniques for identifying COVID-19 cases are much sought after to help reduce transmission – especially given the broad shortages of the gold-standard real-time reverse transcription polymerase chain reaction (rRT-PCR) testing kits. One diagnostic approach that has attracted considerable scientific interest lies in the use of lung CT scans, which can reveal early changes associated with the SARS-CoV-2 virus.
To accelerate the rate at which such scans can be processed, various researchers have turned to deep-learning techniques with a mind to automating image analysis. But it is here that a problem is encountered. Machine-learning tools require huge pre-labelled datasets on which to train themselves, especially if they are to be capable of operating to a clinical standard. Such datasets, however, are not readily available at present – due in part to concerns over patient confidentiality, but also due to the fact that the medical professionals who would be needed to annotate such images are presently occupied in the treatment of patients with COVID-19.
To get around this issue, computer scientists Arpita Halder and Bimal Datta of the Budge Budge Institute of Technology in Kolkata turned instead to transfer learning. In this machine-learning technique, a model developed for one task is then repurposed as a starting point towards tackling a different problem. This reduces the amount of time required for, and the complexity involved in, training of the new system.
In their study, the duo developed a software architecture that they have dubbed KarNet, which they can use on top of four existing deep-learning models: DenseNet201, MobileNet, ResNet50V2 and VGG16. All four models were originally trained to classify 1000 different object categories based on data sourced from ImageNet, an online visual database containing more than 14 million hand-annotated pictures grouped into categories based on what they depict. The team first trained their system on a set of non-modified lung CT images, before moving to using so-called “augmented” images that had been distorted to provide a more realistic challenge.
“We used deep-learning technology for the classification of COVID-19 positive and COVID-19 negative patients effectively,” Halder tells Physics World. “We used four transfer-learning models and concluded that our architecture KarNet on top of DenseNet201 gained a maximum accuracy of 97% with an excellent diagnostic ability having 0.99 AUC [area under the curve] score.”
Robust, fast, adaptable and precise
While DenseNet201 performed the best – a feat Halder attributes to it being a convolutional neural network that is 201 layers deep – the other models fell not far behind, with ResNet50V2, MobileNet and VGG16 achieving accuracies of 96%, 95% and 94%, respectively.
“Despite being trained on the ImageNet dataset, the transfer-learning models have feature-independent benefits of the pre-trained weights, such as scaling and convergence speedups,” Halder explains.
One of the particular benefits of the MobileNet model, Halder says, is that it has a light architecture – meaning that it could easily be deployed onto devices like smartphones and tablets for diagnosis of lung scans in various clinical settings. Given all this, she concludes that the KarNet framework is “robust, fast, adaptable and precise”.

Machine-learning models that detect COVID-19 on chest X-rays are not suitable for clinical use
“Currently, there exists some concern on the efficiency of diagnosis in regional hotspots worldwide and the effectiveness of traditional rRT-PCR working on COVID-19 variants. Hence, research interests in lung CT scan-based AI diagnosis are rising,” comments Yu-Dong Zhang, a machine-learning researcher from the University of Leicester who was not involved in the present study. “The method in this paper does not use the most state-of-art AI methods,” he adds, “but the accuracy on the open-access dataset is rather promising at 97%. I hope that their method can go through strict clinical verification.”
With their initial study complete, the researchers are now looking to move to three-dimensional lung segmentation to facilitate better classification of COVID-19 positive and negative cases. Similarly, they will also be training their deep-learning models on larger datasets in order to secure improvements in diagnostic ability.
The study is described in Machine Learning: Science and Technology.