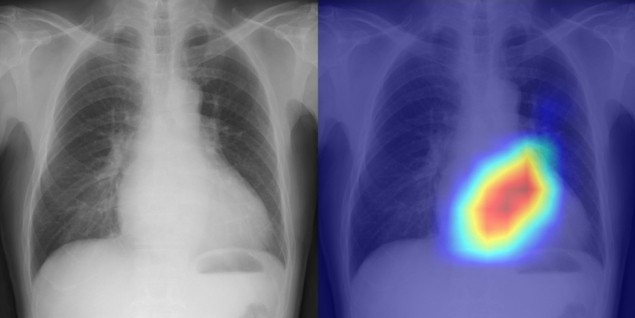
Echocardiography – an ultrasound scan of the heart – is the most frequently used imaging modality for assessing cardiac function and diseases. The technique, however, requires specialized skills that are often in short supply. An alternative option could be to use chest X-rays, one of the most common and widely available medical exams, primarily employed for diagnosis and management of lung diseases. But while the heart is visible in chest radiographs, the relationship between chest radiographs and cardiac health is poorly understood.
Aiming to bridge this gap, a research team led by Daiju Ueda of Osaka Metropolitan University has developed a deep-learning model that uses artificial intelligence to detect valvular disease and classify cardiac function from chest radiographs with unprecedented accuracy. The researchers publish their results in The Lancet Digital Health.
Deep-learning models that are trained and tested on a single dataset can be prone to overfitting, in which the final model only works well for images in the training dataset. To prevent this, Ueda and colleagues developed their model using data from four different institutions, with a total of 22,551 chest radiographs plus associated echocardiograms collected from 16,946 patients.
The researchers used 17,293 radiographs from three institutions to train the deep-learning model, plus 1947 radiographs from the same sites as internal test datasets. For external testing, they employed 3311 radiographs from 2617 patients at a separate institution.
After labelling the chest X-rays using the echocardiography reports as ground truth, the researchers trained their model to learn features connecting the two datasets. They examined six types of valvular heart disease – mitral regurgitation, aortic stenosis, aortic regurgitation, mitral stenosis, tricuspid regurgitation and pulmonary regurgitation – classifying the severity of each disease as none, mild, moderate or severe. They also classified three measures of cardiac function: left ventricular ejection fraction, tricuspid regurgitant velocity and inferior vena cava dilation.
To evaluate the diagnostic performance of their deep-learning model, the researchers calculated the area under the receiver operating characteristic curve (AUC) for nine primary classifiers – a cutoff of none–mild versus moderate–severe for each of the six valvular heart diseases, plus cutoffs of 40% for left ventricular ejection fraction, 2.8 m/s for tricuspid regurgitant velocity, and 21 mm for interior vena cava dilation – for the internal and external test datasets.
The team found that the model could accurately classify cardiac functions and heart diseases, information typically obtained from echocardiography, using information from the chest radiographs. The overall mean AUCs for the primary classifiers were 0.89, 0.90 and 0.92 for the internal test datasets, and 0.87 for the external test dataset (values closer to 1 indicate better classification).
Focusing on the external test dataset, the model could precisely categorize the six types of valvular heart disease, with AUCs ranging from 0.83 to 0.92. The AUC for classifying left ventricular ejection fraction was 0.92, while the AUC for both tricuspid regurgitation velocity and interior vena cava dilation was 0.85.
“To the best of our knowledge, this study is the first to create and validate a deep learning-based classification model for cardiac functions and valvular heart disease using chest radiographs from multiple institutions,” the researchers write.
They point out that the model has several advantages over echocardiography-based evaluation of cardiac disease. Chest X-rays are easy and quick to record and the model can be applied rapidly with low computational requirements. After its initial implementation, the model could be used without any specialized skills and at any time. In addition, it should be possible to use existing chest radiographs to provide information on cardiac function when necessary, without needing additional tests.
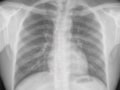
AI model determines cardiovascular risk from routine chest X-ray
“It took us a very long time to get to these results, but I believe this is significant research,” says Ueda in a press statement. “In addition to improving the efficiency of doctors’ diagnoses, the system might also be used in areas where there are no specialists, in night-time emergencies, and for patients who have difficulty undergoing echocardiography.”
“In the future we hope to evaluate the real-world applicability of our model in various clinical settings,” co-author Shannon Walston tells Physics World. “It is crucial for us to understand how our AI-based model can be integrated seamlessly into clinical workflows, and how it can contribute to improved patient care.”